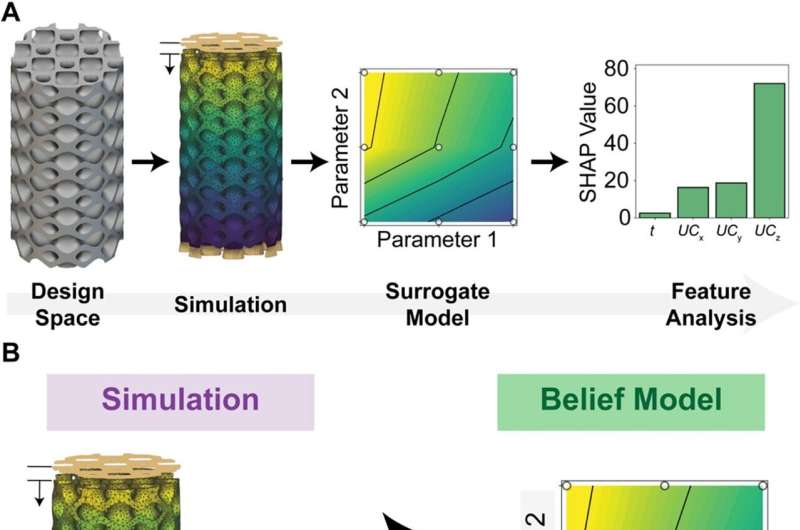
Characterized by their intricate patterns and hierarchical designs, lattice structures hold immense potential for revolutionizing industries ranging from aerospace to biomedical engineering, due to their versatility and customizability. However, the complexity of these structures and the vast design space they encompass have posed significant hurdles for engineers and scientists, and traditional methods of design exploration and optimization often fall short when faced with the sheer magnitude of possibilities within the lattice-design landscape.
Lawrence Livermore National Laboratory (LLNL) scientists and engineers are looking to address these longstanding challenges by incorporating machine learning (ML) and artificial intelligence to accelerate design of lattice structures with properties like low weight and high strength, that can be optimized with unprecedented speed and efficiency.
In a recent study published by Scientific Reports, LLNL researchers fused ML-based approaches with traditional computational techniques in hopes of ushering in a new era in lattice design. By harnessing the power of ML algorithms, researchers are unlocking the ability to predict mechanical performance, optimize design variables and speed up the computational design process for lattices that possess millions of potential design options.
“By leveraging machine learning-based approaches in the design workflow, we can accelerate the design process to truly leverage the design freedom afforded by lattice structures and take advantage of their diverse mechanical properties,” said lead author and LLNL engineer Aldair Gongora.
“This work advances the field of design because it demonstrates a viable way of integrating iterative ML-based approaches in the design workflow and underscores the critical role ML and artificial intelligence (AI) can play in accelerating design processes.”
At the heart of this new research is the development of ML-based surrogate models that serve as virtual prototypes for exploring the mechanical behavior of lattice structures. These surrogate models, trained on a wealth of data incorporating various lattice families and geometric design variables, exhibit remarkable predictive capabilities and can provide invaluable insights into design parameters and the role of geometry and structure on mechanical performance, with an accuracy exceeding 95%, Gongora said.
In addition, by including ML-based approaches in the design loop, the team demonstrated optimal designs could be hastened by exploring less than 1% of the theoretical design space size, he said.
To navigate the vast landscape of lattice design possibilities efficiently, the researchers turned to approaches like Bayesian optimization, a sophisticated form of active learning. By intelligently selecting and evaluating designs in a sequential manner, Bayesian optimization streamlines the exploration process—reducing the number of simulations required to find high-performing designs by five times—and can identify high-performing lattice configurations with extraordinary speed, researchers said.
The approach not only reduces the number of simulations needed to find new designs, but also minimizes the computational burden associated with exhaustive design searches, researchers said.
The team also employed Shapley additive explanation (SHAP) analysis—a method used to understand how different factors or variables contribute to a particular outcome or prediction in a model—to interpret the impact of individual design variables on performance. By dissecting the contributions of each parameter to the overall mechanical behavior, researchers said they could gain a deeper understanding of the intricate relationships within the design space.
Researchers said the study sets a new standard for intelligent design systems—and that the fusion of computational modeling, ML algorithms and advanced optimization techniques represents a leap forward in engineering capabilities that could enhance the performance of aerospace components and revolutionize the field of advanced materials.
Gongora called the work a “critical advancement in demonstrating the various ways AI can play an imperative and beneficial role in materials science and manufacturing,” with an impact extending far beyond the realm of lattice structures.
While the paper focuses on mechanical design, the approach could be applied to a variety of design challenges that rely on expensive simulations, researchers said. Given LLNL’s world-class expertise in additive manufacturing, Gongora said a variety of lattice structures could be physically fabricated, tested and utilized in cross-cutting applications that span the Lab’s mission areas.
“We envision our research being widely implemented in workflows that rely on expensive simulations,” Gongora said. “These ML-based surrogate models could be critical in multi-scale design problems that rely on one or several expensive simulators. Furthermore, we envision our research being used to accelerate parametric design optimization challenges where a scientist, engineer or designer must consider a vast number of design parameters that span both structure and materials.
“By accelerating the computational design process, interesting and novel designs can be intelligently downselected for experimental testing. This creates numerous opportunities for scientists to use ML tools in their research and design challenges in the sciences.”
LLNL co-authors included Caleb Friedman, Deirdre Newton, Timothy Yee, Zachary Doorenbos, Brian Giera, Eric Duoss, Thomas Y.-J. Han, Kyle Sullivan and Jennifer Rodriguez.
More information:
Aldair E. Gongora et al, Accelerating the design of lattice structures using machine learning, Scientific Reports (2024). DOI: 10.1038/s41598-024-63204-7
Citation:
Researchers unleash machine learning in designing advanced lattice structures (2024, August 22)
retrieved 23 August 2024
from https://techxplore.com/news/2024-08-unleash-machine-advanced-lattice.html
This document is subject to copyright. Apart from any fair dealing for the purpose of private study or research, no
part may be reproduced without the written permission. The content is provided for information purposes only.